The Future of Agriculture: AI-Powered Hyperspectral Imaging for Sorghum Nutrient Detection
- Dr. Julie Butenko
- Feb 25
- 4 min read
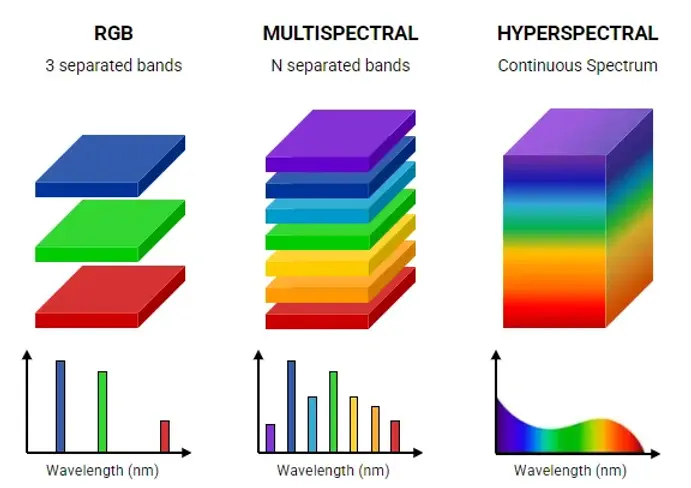
The global agricultural industry is undergoing a paradigm shift with the integration of artificial intelligence (AI) and hyperspectral imaging (HSI) technologies. These advancements offer unprecedented accuracy and efficiency in crop quality assessment, addressing challenges such as food security, nutrient optimization, and large-scale crop analysis.
One of the most promising breakthroughs in this field is the use of visible and near-infrared (VIS-NIR) hyperspectral imaging combined with machine learning to detect the nutrient composition of sorghum, a staple cereal crop cultivated worldwide.
Traditional chemical-based nutrient analysis methods—though reliable—are often time-consuming, destructive, and expensive. Hyperspectral imaging, augmented by AI-driven algorithms, is changing the landscape by enabling real-time, non-destructive, and high-throughput nutrient detection.
This article explores the science, technology, applications, and future implications of hyperspectral imaging in sorghum nutrient assessment and beyond.
Sorghum: An Essential Global Crop
Sorghum (Sorghum bicolor) is the fifth most important cereal crop globally, following maize, wheat, rice, and barley. It is cultivated primarily in Africa, Asia, and the Americas, thriving in regions where other crops struggle due to drought tolerance and low water requirements.
Nutritional and Economic Significance
Nutrient | Function in Human and Animal Diets | Content Range (%) in Sorghum |
Crude Protein | Essential for growth, enzyme function, and metabolism | 7-15% |
Crude Fat | Provides energy, essential fatty acids | 1.5-6% |
Tannin | Antioxidant properties, but excessive amounts reduce digestibility | 0.1-3% |
The variation in nutrient content between sorghum varieties poses challenges for breeders, farmers, and industries in selecting optimal grains for food, feed, and biofuel production.
Traditional Methods vs. Hyperspectral Imaging
Limitations of Conventional Nutrient Detection Methods
Historically, nutrient analysis in grains has relied on wet chemistry techniques, such as:
Kjeldahl method for crude protein determination
Soxhlet extraction for fat analysis
Spectrophotometry for tannin quantification
These methods, while accurate, suffer from several drawbacks:
Factor | Traditional Chemical Methods | Hyperspectral Imaging (HSI) |
Time Required | Hours to days | Seconds to minutes |
Destructiveness | Destroys samples | Non-destructive |
Cost | High (chemicals, equipment, labor) | Lower after initial investment |
Scalability | Limited to lab conditions | Field-ready, real-time application |
Hyperspectral imaging, in combination with machine learning algorithms, offers a non-invasive, rapid, and cost-effective alternative, paving the way for a new era of precision agriculture.
How Hyperspectral Imaging and AI Work Together
The Science Behind Hyperspectral Imaging
Hyperspectral imaging captures a wide range of spectral information beyond the visible spectrum (400-700 nm). It extends into the near-infrared (NIR, 700-2500 nm) region, where molecular interactions of proteins, lipids, and polyphenols can be detected.
The working principle of HSI involves:
Illumination: A light source irradiates the sorghum samples.
Spectral Acquisition: The sensor captures reflectance data across multiple wavelengths.
Data Processing: Machine learning algorithms analyze spectral signatures to predict nutrient composition.
Machine Learning Models for Sorghum Analysis
A study conducted at Shanxi Agricultural University analyzed 279 sorghum samples from 93 varieties, using advanced AI algorithms:
Algorithm | Function | Accuracy (Rp² Value) |
Partial Least Squares Regression (PLSR) | Linear correlation between spectra and nutrients | 0.78-0.85 |
Backpropagation Neural Network (BPNN) | Deep learning-based feature extraction | 0.87-0.92 |
Extreme Learning Machine (ELM) | Fast, high-accuracy prediction model | 0.93-0.97 |
Among these, the ELM model combined with Competitive Adaptive Reweighted Sampling (CARS) and Bootstrapping Soft Shrinkage (BOSS) achieved the best results, accurately predicting crude protein, fat, and tannin contents.
Key Spectral Ranges for Nutrient Detection
Nutrient | Wavelength Range (nm) | Correlation with Nutrient Composition |
Crude Protein | 434–899 | Absorption due to amino acid bonds |
Crude Fat | 447–900 | Lipid bond vibrations |
Tannin | 475–897 | Polyphenolic compound absorption |
Real-World Applications and Industry Impact
Precision Agriculture
Farmers can use handheld hyperspectral sensors to assess grain quality before harvesting, optimizing yield and quality.
Food and Feed Quality Assurance
Food manufacturers and animal feed producers can rapidly screen sorghum batches, ensuring consistent nutritional standards.
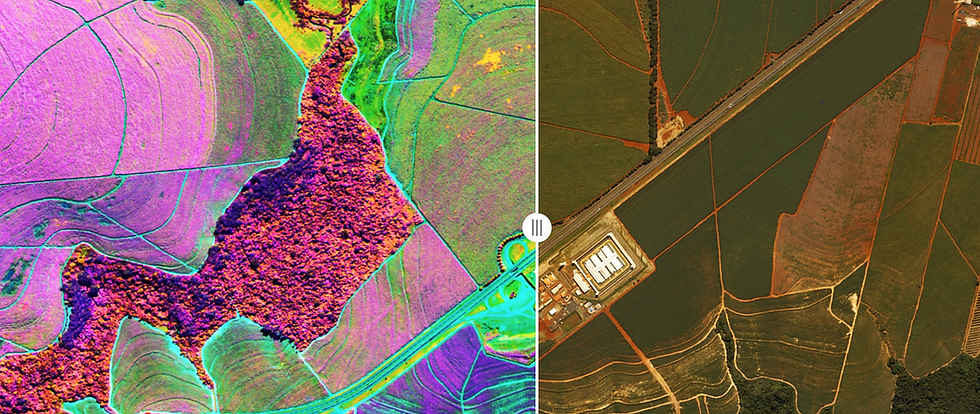
AI-Driven Supply Chain Optimization
AI can integrate hyperspectral data into big data platforms, helping suppliers identify high-quality grains for specific applications, reducing waste and maximizing efficiency.
Real-Time Crop Monitoring
Drones equipped with hyperspectral cameras can scan entire fields, detecting nutrient deficiencies and optimizing fertilizer use, reducing costs and improving sustainability.
Future Prospects: Integrating AI, Big Data, and Automation
With advances in deep learning and real-time data analytics, future systems could:
Enable real-time grain sorting at industrial processing plants.
Integrate with blockchain technology to ensure transparent quality tracking.
Use AI-powered drones for real-time soil and crop health assessments.
Expert Insights
According to the study’s lead researchers:
“These detection models effectively achieved real-time and non-destructive detection of crude protein, tannin, and crude fat contents in sorghum grains, revolutionizing the way agricultural industries assess quality.”
Such advancements promise to streamline agricultural processes, ensuring a secure and sustainable food supply for the future.
The Role of AI in Transforming Agriculture
The fusion of hyperspectral imaging and AI is reshaping agricultural science, enabling faster, cheaper, and more accurate nutrient assessment. This technology holds immense potential beyond sorghum, with applications in wheat, rice, maize, and other staple crops.
As AI continues to evolve, companies and researchers must work together to harness data-driven insights for improving food security, sustainability, and global agricultural productivity.
For more expert insights on AI, predictive analytics, and emerging agricultural technologies, follow Dr. Shahid Masood and the expert team at 1950.ai. Stay ahead with the latest breakthroughs in AI-driven precision farming and crop monitoring.
Comments