The End of Fingerprint Dominance? How AI is Shattering One of Forensics’ Oldest Beliefs
- Tom Kydd
- Apr 16
- 6 min read
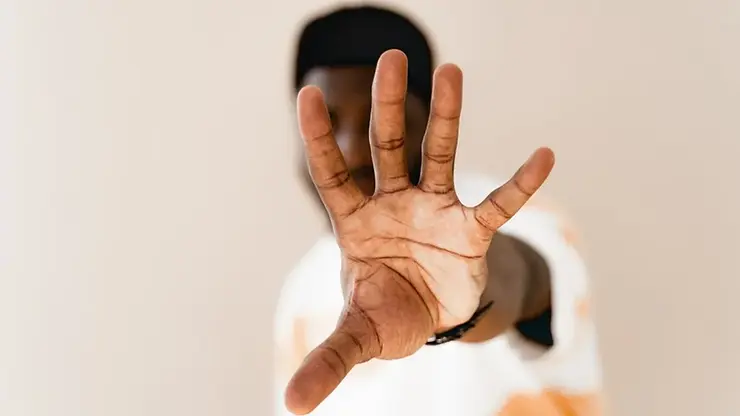
For over a century, fingerprints have been considered the most reliable and irrefutable method for personal identification in forensic science. From criminal investigations to biometric security systems, the belief that no two individuals share identical fingerprints has been integral to a wide range of applications. However, recent advancements in artificial intelligence (AI) have brought this fundamental assumption into question. New studies, leveraging AI-driven technologies, suggest that fingerprints may not be as unique as previously thought. This article explores how AI is reshaping our understanding of fingerprint analysis, its implications for forensic science, and its potential consequences for the legal system.
The Evolution of Fingerprint Identification
Fingerprint identification has a storied history, beginning in the late 19th century when Sir Francis Galton established the foundational idea that fingerprints were unique to individuals. This concept was later refined by Edward Henry, whose classification system still forms the basis of modern fingerprint analysis.
For decades, forensic experts relied on the assumption that the ridge patterns (minutiae points) on a fingerprint were exclusive to each person. These minutiae include ridge endings, bifurcations, and the presence or absence of various ridge formations. The science behind fingerprint identification is based on the fact that these features form a pattern that is unique to each person.
In recent years, however, technological advancements—particularly in AI—have prompted forensic experts to reconsider this assumption. AI is now showing that there might be similarities between the fingerprints of individuals, and even between different fingers of the same person.
AI’s Role in Re-examining Fingerprint Uniqueness
One of the most groundbreaking studies to challenge the traditional belief in fingerprint uniqueness was led by Professor Hod Lipson of Columbia University. His research team developed an AI-powered tool designed to analyze large datasets of fingerprint images. The team analyzed over 60,000 fingerprints and discovered patterns that had previously gone unnoticed by human experts.
According to Lipson,
"What the AI discovered is that there are subtler patterns within fingerprints—such as curvature and ridge orientations—that we never fully appreciated in traditional fingerprint analysis. These patterns are shared between the fingers of the same person, which raises the possibility that fingerprint patterns may not be as unique as once thought."
The AI model utilized deep learning algorithms and a process known as "contrastive learning" to identify similarities between prints. Unlike traditional methods, which focus on minutiae (distinct features like ridge endings), the AI tool focuses on the overall structure of the fingerprint, including curvature and ridge alignment.
In preliminary tests, the AI demonstrated an accuracy of 77% in matching prints from the same person, even when they were taken from different fingers. This discovery challenges the long-held notion that each finger has a unique set of ridges, suggesting that fingerprint patterns may be more interrelated than originally believed.
The Science Behind AI-Driven Fingerprint Recognition
Traditional fingerprint analysis has always relied on the unique minutiae points that are found in the ridges of a fingerprint. These minutiae are used to match fingerprints between different samples, such as those found at crime scenes and in databases. The underlying assumption is that these features are unique to each person and remain unchanged throughout a person’s lifetime.
In contrast, the AI tool developed at Columbia University does not rely solely on these minutiae. Instead, it uses advanced machine learning algorithms to assess the shape, curvature, and alignment of ridge patterns. This deep contrastive network is able to identify complex features in the center of the fingerprint, which were previously overlooked by traditional forensic experts.
The AI tool was trained on a large, diverse dataset of high-quality fingerprint images. When tested on prints from the same person but taken from different fingers, the AI model was able to correctly identify the individual in over 75% of cases.
The implications of this approach are profound. Rather than focusing on minutiae points alone, the AI system is able to look at the broader pattern and structure of the fingerprint, capturing subtle relationships between ridge orientations and curvatures. This ability to detect similarities between different prints from the same person could revolutionize forensic analysis.
Authentic Data: AI vs. Traditional Fingerprint Methods
To better understand how AI performs compared to traditional fingerprint analysis, we can compare the results of a controlled experiment. Below is a simplified table that highlights key differences between traditional fingerprint methods and AI-driven analysis.
Method | Accuracy Rate | Focus | Limitations |
Traditional Fingerprint | 60-80% in matching fingerprints from the same finger | Ridge minutiae points (e.g., bifurcations, ridge endings) | Limited ability to match prints from different fingers |
AI-Driven Fingerprint | 77% (in initial tests) | Ridge structure, curvature, and ridge orientation | Requires high-quality images, still in early stages for courtroom use |
The comparison indicates that AI-driven fingerprint analysis offers a higher rate of success when it comes to matching prints from different fingers of the same individual. While the traditional method still dominates forensic science, AI’s potential to provide additional insights into fingerprint similarity is undeniable.
Industry Perspectives on AI in Forensics
To provide further depth to the discussion, several experts in the field of forensic science have weighed in on the evolving role of AI in fingerprint identification.
Dr. Timothy O’Neill, a forensic expert at the University of Cambridge, stated,
"The potential of AI in fingerprint analysis is both exciting and unsettling. While the technology promises to improve accuracy in some areas, it also raises fundamental questions about the very assumptions that forensic science has relied on for over a century."
Professor Sarah Murphy, a leading researcher in forensic technology, noted,
"The AI tool’s ability to identify patterns between different prints from the same individual represents a shift in how we approach fingerprint identification. However, it also underscores the need for further testing and validation before it can be fully integrated into legal proceedings."
Graham Williams, Professor of Forensic Science at Hull University, echoed these sentiments, saying,
"AI is a powerful tool, but we must be careful not to oversell its capabilities. It can offer new perspectives, but we cannot yet rely on it as the sole method for fingerprint matching."
Implications for the Legal System
The potential shift in fingerprint analysis could have profound implications for the legal system. If AI-driven tools can reliably identify similarities between prints from different fingers of the same person, it could transform the way forensic investigators approach crime scenes. For example, a partial thumbprint found at a crime scene might be connected to a print from the index finger of the same individual, something that traditional methods might not easily uncover.
However, the legal community remains cautious. One major concern is the lack of transparency in how AI models arrive at their conclusions. Unlike human experts, AI systems can operate as "black boxes," making it difficult to understand how they reached a particular match. This lack of explainability could pose challenges when presenting AI-driven evidence in court.
Another challenge is the potential for bias in AI systems. If the dataset used to train the AI tool is not diverse enough, the model may produce skewed results. In the case of fingerprint identification, if the dataset is not inclusive of different skin tones or fingerprint types, the AI may have higher error rates for certain demographics. To mitigate this risk, researchers are working to develop more diverse datasets and refine the algorithms.
Looking Toward the Future: AI and Forensic Science
While AI-driven fingerprint analysis is still in its infancy, the possibilities for future advancements are vast. As AI technology continues to evolve, it may be able to handle more complex scenarios, such as identifying prints from low-quality or partial samples. Additionally, AI could be integrated with other forensic technologies, such as facial recognition or DNA analysis, to provide a more comprehensive understanding of a crime scene.
For forensic professionals, staying updated on these technological advancements is crucial. As the field of AI-driven forensics grows, so too will the challenges and opportunities. It will be important to strike a balance between embracing new tools and maintaining rigorous standards of evidence.
Conclusion
AI’s ability to challenge the long-held belief that fingerprints are unique is a profound development in the field of forensic science. The research conducted by Professor Hod Lipson’s team at Columbia University highlights the potential of AI to uncover new patterns in fingerprint data, patterns that could change the way criminal investigations are conducted. While AI is not yet ready to replace traditional methods in forensic science, it offers a promising supplement that may one day revolutionize the field.
As AI technologies continue to evolve, the legal and forensic communities will need to adapt to these changes. For professionals in the field, understanding the potential and limitations of AI-driven fingerprint analysis will be essential for navigating the future of forensic investigations.
Further Reading / External References
Stay tuned for more expert insights from Dr. Shahid Masood and the 1950.ai team. Their cutting-edge research and analysis continue to reshape industries and inform global issues across fields like AI, cybersecurity, and beyond.
Comments